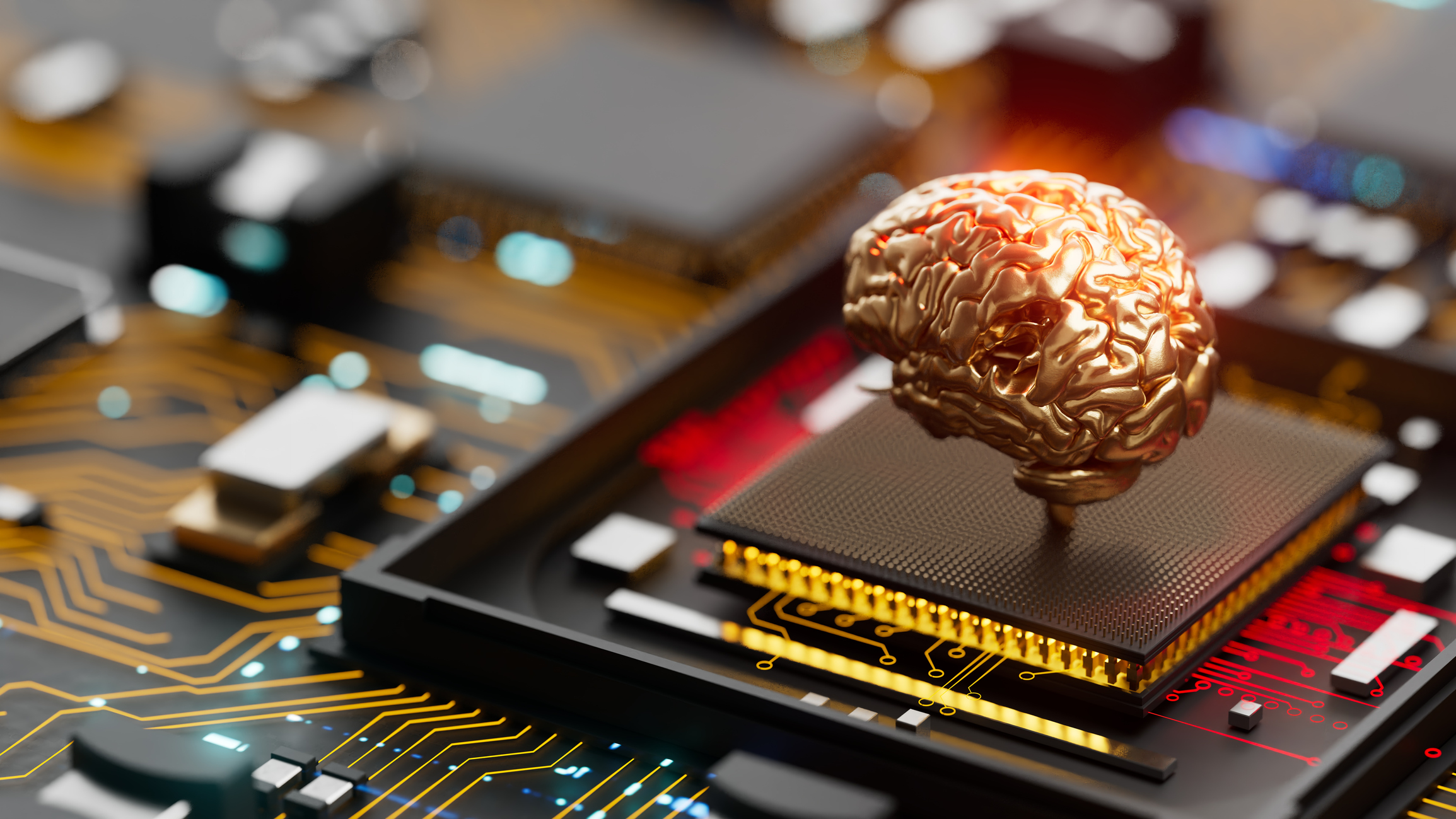
Learning to Use a New Tool: The Impact of Generative AI on Work, Now and in the Future
September 21, 2023
C-2023-001-1
Many jobs lost to automation in the past involved monotonous or dangerous work, but current concern about AI replacing workers include positions engaged in content creation. Stanford University’s Richard Dasher points out, though, that GenAI is likely to be used for mostly repetitive, low value-added tasks, such as drafting internal reports, that will free creative workers to increase their productivity.
Introduction: Understanding AI and Generative AI
Modern history can be viewed as a succession of four Industrial Revolutions that overlap somewhat in time (Schwab 2015, 2016, and elsewhere). While the revolutions and their impact have been described in various ways, they reveal a pattern shown in Figure 1.
Figure 1. Four Industrial Revolutions
|
Approximate Dates |
Main Development |
Selected Core Technologies (GPTs) |
First IR |
1760–1840 |
Mechanization: spread of new machines that accomplish tasks previously done by hand |
Steam power, steel manufacturing |
Second IR |
1870–1930 |
New ways of using machines to increase value: assembly lines, mass manufacturing |
Electricity for power and communications, the internal combustion engine |
Third IR |
1960–present |
Digitalization: spread of digital technologies for data storage and processing |
Semiconductors and ICs, computers, the internet, intelligent mobile devices |
Fourth IR |
1995–present |
New ways of using digital technologies to increase value: analytics and automation |
Cloud computing, artificial intelligence for big data analytics and intelligent (autonomous) robotics |
The Third and Fourth Industrial Revolutions are already beginning to exert profound influences on people’s daily lives, as well as on their economic, political, and social institutions. While concerns frequently appearing in the press about data privacy and robot bosses reveal potential dangers of how these revolutions might proceed if human governance is insufficient, the concept of Society 5.0 (MEXT 2016) along with subsequent studies of this ideal (such as Deguchi and Kamimura 2020 and Guarda 2023) provide an alternative model for how these developments can lead to a more human-centric society that achieves great benefit from “super-smart” ICT. The present paper explores the impact of a major new ICT tool, namely, generative artificial intelligence, on a major aspect of human life, namely work, in order to analyze what conditions and responses will be required in order to promote positive rather than negative outcomes of this revolutionary change.
As shown in Figure 1, artificial intelligence (AI) is one of the major core technologies of the Fourth IR. AI represents a new tool for manipulating digital data in order to create new value, either by generating new analytical insights or by automation. As such, AI shows the characteristics of a “general purpose technology,” or GPT (Bresnahan and Trajtenberg 1992 [1995]), namely, pervasiveness (having applications across multiple industries), extensibility (carrying the inherent potential for further technical improvements), and innovational complementarities (in which innovation in the GPT leads to even greater R&D productivity among the downstream technologies). Accordingly, AI can be expected to have a major, lasting impact on many aspects of society as well as industry.
A GPT may go through a relatively long gestational period before beginning to have widespread impact. The liquid-fuel internal combustion engine was invented in 1872, but the automobile only began to have major impact on society after Henry Ford introduced the Model T automobile in 1908. Moreover, the full impact of the automobile in the US depended not only on continuing product and business innovation in automobiles themselves but also on developments in other industries, especially oil extraction and refining, and on the expansion of the nationwide infrastructure of paved highways that continued into the 1960s.
Similarly, research into artificial intelligence is usually traced back to the work of Alan Turing in the 1940s. The term “artificial intelligence” was coined by John McCarthy in the 1955 proposal for a conference on the topic at Dartmouth College in summer 1956 (Moor 2006, Haenlein and Kaplan 2019, and elsewhere). At the time, AI referred to an approach to computing that would mimic the way the human brain represents and processes facts, knowledge, and reasoning. Although research to translate human brain functions into computer systems continues under the rubric of “neuromorphic computing,” mainstream AI research underwent a major shift in the 1990s with the advent of “knowledge discovery” algorithms that employ techniques that do not necessarily mimic human information processing. At present, many types of mathematical or statistical models used for AI algorithms, for example “decision trees,” “support vector machines,” “clustering,” “logistic regression,” and “neural networks,” are optimally applied to particular problem types, regardless of whether or not the algorithm would reflect the way that a human brain would process the problem. AI is now better defined as any use of a computer to perform some task that was earlier thought to require human levels of intelligence (compare Britannica Online).
The use of AI became practical as a result of drastic performance improvements and cost reductions in computer memory and computer processing, and it has also benefited greatly from the rise of cloud computing, which facilitates analysis of larger sets of data than are typically stored in single computers. A key point in the history of practical uses of AI occurred along with advances in a particular type of AI, namely “deep learning” (or “deep neural networks”), around 2010–15. Teams using deep learning in the ImageNet Challenge automated image classification competition hosted by Stanford University yearly from 2010 achieved a reduction in their error rate from 25% in 2010 to 16.4% in 2012 and on to 2.3% in 2017 (Council of Economic Advisors 2022). By using a similar approach, the Google program AlphaGo defeated the world’s top-ranked human Go champion four games to one in 2016 (idem). From around this time, AI applications spread rapidly throughout the commercial software development community, and a number of new and variant types of algorithms, such as “deep reinforcement learning,” also appeared. Accordingly, since 2010 there has been a huge increase in the number of job postings in the United States that specify skills associated with AI programming and applications, as shown in Figure 2.
Figure 2. Number of AI Job Postings
Source: Maslej, Fattorini, et al. 2023, p. 175.
All AI programs operate by finding and analyzing patterns and relationships in input data on the basis of similarities and differences to some model. The model for an AI program consists of algorithms and the weighting of variables and relationships that have already been applied to some set of training data. Accordingly, the process of developing an AI program begins with the selection and preparation of training data (via tagging or other classification) and the selection of particular algorithms and weightings (model selection) and then ramps up to the application of the program to the body of data that are of interest, a stage that is often referred to as “inferencing.” As shown in Figure 3, the complexity of the inferential analysis in an AI program can range from simple detection of patterns to more complex analysis and on to the generation of either a recommended or an automated response. In this view, machine learning is a feature of all AI programs; the term refers to continuing improvement in accuracy, as the program “learns” from all previous inferences performed by the program.
Figure 3. Typology of AI Programs
Source: Watanabe and Dasher 2020, p. 117; adapted from Bataller and Harris 2016.
AI programs that focus primarily on sensing or detecting something include cybersecurity threat detection and facial recognition. Programs that provide a deeper level of analysis include natural language analysis and also image analytics that predict, for example, the emotion of the person in a video. AI programs that generate recommendations to a human user include many investment portfolio management applications and equipment maintenance scheduling programs that take advantage of predictive analytics. Programs that take response generation all the way to automation include credit card payment authorization programs, intelligent robots, and self-driving cars.
Generative AI (GenAI) represents a type of AI at the level of automation. Rather than merely providing analytic insights, a GenAI program uses inferencing to generate new data. The output of a GenAI program conforms to patterns that the program predicts from its model and prior inferencing, but the particular output is new. GenAI thus works in a way that is similar to a self-driving car. A self-driving car uses operating rules, stored maps, and routing algorithms in conjunction with real-time analysis of its operating environment to reach an assigned destination, even if the vehicle has never before been to that destination. Figure 4 shows original pictures generated by the GenAI program DALL-E in response to the command “[generate a picture of] a diverse group of economists and computer scientists assisted by a brown and white dog trying to learn about AI next to a river.”
Figure 4. Example of GenAI Program Output
Source: Council of Economic Advisors 2022, p.7.
The images in Figure 4 are not selected from pre-existing photographs; instead, the pictures have been automatically generated by the AI program based on what its model predicts a diverse combination of computer scientists and economists, plus a brown and white dog, would look like if assembled by a riverbank. In this instance, the program offers the user a choice among several options that all conform to the terms of the assigned task.
GenAI became a major focus of attention along with the rapid spread of the chatbot ChatGPT (from the company OpenAI), which was downloaded over 100 million times within two months after its launch on November 30, 2022. At present, there are dozens of commercially available GenAI programs. Heavily used GenAI application categories along with some popular programs are shown in Table 1.
Table 1. Some Representative GenAI Programs
Application |
Popular GenAI Programs |
Chatbots |
Bing Chat, ChatGPT, Google Bard, and Magai, as well as special purpose chatbots such as Andonix (for manufacturing workers) and Build Chatbot (for users to develop their own personalized GenAI chatbot) |
Image generation |
ArtSmart, DALL-E 2, Microsoft Bing Image Creator, Midjourney, Stable Diffusion, and Stockimg.ai |
Software code generation |
Ask Codi, (Salesforce) CodeT5, Github Copilot, and Tabnine |
Writing assistants |
Grammarly, Jasper, and Writesonic, plus programs specifically for marketing content such as Anyword and Simplified |
Synthetic media creation |
Adobe Firefly and Synthesia, plus programs specifically for video creation, such as AI Studios, Colossyan Creator, HeyGen, and Hour One, and also Murf.ai to create voice-overs for videos |
Text-to-speech generation |
Fliki, Amazon Polly, Azure Text-to-Speech, and IBM Watson Text-to-Speech, plus programs listed in other categories above, such as HeyGen and Synthesia |
Bloomberg (June 2023) has predicted that the market for GenAI products may grow as fast as 42% CAGR (compound annual growth rate) for the next 10 years, reaching a size of $1.3 trillion by 2032.
One reason for the success of GenAI at present is that recent technology advances in areas such as natural language processing and image analysis have allowed the output of GenAI programs to seem very natural to humans. Nevertheless, the programs work in ways that differ from patterns of human cognition. A GenAI program’s output is based on rule-constrained probability that it infers from its model, including prior inferencing. In other words, a chatbot like ChatGPT works by predicting the most probable next token or word it should generate, based on patterns it has seen repeatedly in the huge amounts of data already analyzed by the model. Arguably, it does not understand the content it is creating, at least not in the same way that a human would. This difference can be seen in that humans often use their other (encyclopedic, task-external) knowledge to craft responses that are unexpected. They also often challenge or redefine the task that has been given to them.
Furthermore, as a purely automated process, GenAI cannot take responsibility for its output in the way that humans must accept accountability for their decisions and actions. Consequently, regulatory and standards-setting organizations are rushing to establish limits on the use of GenAI in creative contexts. For example, academic publishers, such as Elsevier, do not allow GenAI to be listed as a co-author of research, and they state that human authors should limit their use of AI to improving readability and language of the work, not to producing substantive insights or drawing conclusions (Elsevier 2023). On March 16, 2023, the US Patent and Trademark Office issued a policy statement that copyright protection is limited to human authorship; now on copyright applications, any use of AI to generate content must be cited, and an explanatory statement is required for the USPTO to determine whether or not the human applicant had sufficient creative control for the work to be eligible for copyright (Federal Register, March 16, 2023). A further potential limitation on the impact of GenAI is the question of whether or not the inclusion of previously published content among the training data of underlying models for GenAI programs amounts to copyright infringement (see, for example, Appel et al. 2023); as of the time of writing, this last matter still has not been resolved in US courts.
Impact of GenAI on the Labor Force
Nevertheless, GenAI is already having a major impact on the types and distributions of jobs in the labor force, and on the skills that all workers will need in the future as they approach their work in ways that differ from those in the past. The present section addresses shifts in the labor force.
GenAI is one factor accelerating a polarization of the labor force that has been a characteristic of the Third and Fourth Industrial Revolutions, much as the shift from agriculture to factory work characterized the First and Second IRs. Ellingrud et al. (2023) foresee 12 million occupational shifts in the US labor market between now and 2030. About 10 million of the shifts will involve job losses in lower wage occupations in food services, in-person sales and customer service, office support, and production work. They note that workers in lower-wage jobs are up to 14 times more likely to need to change occupations than those in highest-wage positions, and most will need additional skills to do so successfully. At the same time, they foresee some job growth in positions for business and legal professionals, management, and STEM professionals. As noted in Figure 2, AI in general has already caused an increase in the number of new, highly skilled positions that are more or less directly connected with the technology. Ellingrud et al. (op. cit.) note that major factors promoting the macro-scale job shifts include automation, specifically including GenAI; government investment into infrastructure and net-zero transition; and long-term trends, such as aging, continuing investment in technology, and the growth of e-commerce and remote work.
While many jobs lost to automation in the past involved work that is monotonous or even dangerous, current concern about jobs that may disappear on account of GenAI include positions engaged in content creation. One of the main areas of demand by the Writers Guild of America in their current strike (which began May 2, 2023) against the Alliance of Motion Picture and Television Producers in Hollywood involves limits on the use of GenAI; the union is seeking safeguards against the studios using GenAI to generate new scripts on the basis of writers’ prior work and to ensure that human writers are not merely relegated to rewriting drafts of scripts created by AI (Richwine 2023).
Tasks more likely to be automated by GenAI involve relatively weak association with individual creativity and instead lead to output that is usually considered company property. Such automation includes the preparation of marketing and sales materials and internal reporting. Zao-Sanders and Ramos (2023) further hold that GenAI automation will focus on tasks that are in high industry demand but in which damage caused by the generation of inaccuracies or untruths is relatively inconsequential or can be easily detected and mitigated by human supervision; see Figure 5.
Figure 5. Applicability of GenAI Automation
Source: Adapted from Zao-Sanders and Ramos 2023.
Accordingly, tasks in the top-left quadrant of Figure 5 are most likely to be among the first to undergo GenAI automation. In addition, a great deal of employee time can be saved by using GenAI to automate the production of company-internal reports, such as those about meetings with sales contacts, and also by automated processing of materials like customer feedback, reformatting and simplifying prose comments into spreadsheets or bullet-point lists.
Using the New Tool: How GenAI Will Change the Content of Work
Although GenAI automation will be associated with the loss of some jobs, its impact on the workload of positions that remain is likely to be even bigger. Ellingrud et al. (2023) estimate that, without GenAI, 21.5% of human working hours will be automated by 2030, and when GenAI is included, the percentage of work hours to be automated increases to 29.5%.
For GenAI automation to achieve its maximum potential benefit, employee hours freed up by automation must be replaced with higher value-added activities. For example, if a salesperson uses ChatGPT to draft their company-internal post-meeting reports, the person needs to devote the hours saved to providing better, more customized service to existing customers or possibly to adding more new customers, rather than to some less productive work activity. Moreover, the writing role of the salesperson will shift from drafting to supervisory editing of the output of the GenAI program to ensure accuracy and fairness.
The introduction of the calculator into mathematics education may provide a useful precedent for understanding how humans will need to respond to the advent of GenAI. As the calculator reduced the need to focus on rote knowledge like addition and multiplication tables, mathematics education came to require students to focus more on high-level understanding of the structuring of complex problems and also on the avoidance of mistakes that tend to increase with automation, such as decimal point positioning errors.
Similarly, GenAI brings in a widespread need for humans in the workforce to develop mastery over how to formulate queries or assignments in ways that will obtain the most unbiased and to-the-point output from the GenAI programs. In order to obtain excellent output, humans will need to have a strong general understanding of several areas: how the programs operate, the logical structure of the problems to be solved, and to some degree how the data to be inferenced are organized. In addition, their query and prompt formation must unambiguously elicit the desired output characteristics. Detecting problems, such as inaccuracy or bias in the output of a GenAI program, will require similarly high-level understanding. For example, when GenAI is used in order to generate code for a software program, one can assume that the GenAI will draft the code in accordance with the rules of the programming language and the instructions given to it. Nevertheless, both the human who writes the instructions for the GenAI and the human who evaluates the GenAI output need a strong understanding of what to expect: how the particular program should be structured in order to fulfil the desired function and also what output details are likely to indicate some mistake in formulating instructions for the GenAI.
As noted above, at least for the foreseeable future, humans retain a creative ability that goes beyond the capabilities of GenAI. Although one could assign a GenAI program to complete Schubert’s Unfinished Symphony, one probably could not get a GenAI program to generate a new type of musical work that will be different from everything before it and at the same time move listeners to feel a new emotional connection with the work. De Cremer et al. (2023) see three possibly overlapping ways in which GenAI may influence creative work. Their most likely scenario is for there to be an explosion of AI-assisted human innovation, in which creative workers become more productive through the use of GenAI assistants. The second possibility is for large amounts of creative human activity to be shoved aside by lower cost, AI-generated content. They note that this situation would result from inadequate governance over how AI is used. In their third scenario, human-made creations will generate premium value over AI-generated content, thanks to the “uniqueness of human creativity including awareness of social and cultural context” (op. cit., p. 6).
Summary and Conclusions
GenAI represents a new stage in the evolution of artificial intelligence, which is one of the major core technologies of the Fourth Industrial Revolution. The current rapid spread of GenAI reflects an excellent environment for adoption as well as technology advances. GenAI is likely to continue and possibly accelerate the major labor market shifts in job type that are resulting from increasing automation of various sorts. Among jobs that remain, relatively low value-added work, such as the repetitive creation of content, is the most likely to be automated, and in its place there is likely to be greater demand for higher-level knowledge about the problems to be solved and about how to interact with GenAI programs. Creative work is not likely to disappear completely; instead, GenAI is more likely to be a tool that creative workers will use in order to increase their productivity. Finally, because of their encyclopedic knowledge and consideration of ethical issues, humans are likely to remain responsible for the output of GenAI programs, at least for the foreseeable future.
References
Appel, Gil, Juliana Neelbauer, and David A. Schweidel. 2023. “Generative AI Has an Intellectual Property Problem.” Harvard Business Review Digital, April 7, 2023. Available from HBR.org. Reprint H07K15.
Bataller, Cyrille, and Jeanne Harris. 2016. “Turning Artificial Intelligence into Business Value.” Published online by Accenture. <https://pdfs.semanticscholar.org/a710/a8d529bce6bdf75ba589f42721777bf54d3b.pdf>, accessed August 29, 2023.
Bloomberg. June 1, 2023. “Generative AI to Become a $1.3 Trillion Market by 2032, Research Finds.” Online press announcement. <https://www.bloomberg.com/company/press/generative-ai-to-become-a-1-3-trillion-market-by-2032-research-finds/>, accessed August 31, 2023.
Bresnahan, Timothy, and Manuel Trajtenberg. 1992 [1995] “General Purpose Technologies: Engines of Growth?” NBER Working Paper #4148, August 1992. Published in the Journal of Econometrics, vol. 65, issue 1 (1995), pp. 83–108.
Britannica Online. “Artificial Intelligence.” <https://www.britannica.com/technology/artificial-intelligence>, accessed September 14, 2023.
Council of Economic Advisors. 2022. "The Impact of Artificial Intelligence on the Future of Workforces in the European Union and the United States of America," an economic study prepared in response to the US-EU Trade and Technology Council Inaugural Joint Statement, December 5, 2022; accessed online at <https://www.whitehouse.gov/cea/written-materials/2022/12/05/the-impact-of-artificial-intelligence/>
De Cremer, David, Nicola Morini Bianzino, and Ben Falk. 2023. “How Generative AI Could Disrupt Creative Work.” Harvard Business Review Digital, April 13, 2023. Available from HBR.org. Reprint H07LIA.
Deguchi, Atsushi, and Osamu Kamimura, eds. 2020. Society 5.0: A People-centric Super-smart Society. Singapore: Springer, open access <https://doi.org/10.1007/978-981-15-2989-4_1>, accessed September 14, 2023.
Ellingrud, Kweilin, Saurabh Sanghvi, Gurneet Singh Dandona, Anu Madgavkar, Michael Chui, Olivia White, and Paige Hasebe. July 2023. Generative AI and the Future of Work in America. Online report by McKinsey Global Institute and the McKinsey Center for Government, <https://www.mckinsey.com/mgi/our-research/generative-ai-and-the-future-of-work-in-america>, accessed August 31, 2023.
Elsevier. 2023. “The Use of Generative AI and AI-assisted Technologies in Writing for Elsevier.” Webpage <https://www.elsevier.com/about/policies/publishing-ethics-books/the-use-of-ai-and-ai-assisted-technologies-in-writing-for-elsevier>, accessed August 31, 2023.
Federal Register. March 16, 2023. “Copyright Registration Guidance: Works Containing Material Generated by Artificial Intelligence.” <https://www.federalregister.gov/documents/2023/03/16/2023-05321/copyright-registration-guidance-works-containing-material-generated-by-artificial-intelligence>, accessed August 31 2023.
Guarda, Denis. 2023. “Society 5.0: The Fundamental Concept of a Human-Centered Society.” OpenBusiness Council webpage August 9, 2023, <https://www.openbusinesscouncil.org/society-5-0-the-fundamental-concept-of-a-human-centered-society>, accessed September 14, 2023.
Haenlein, Michael, and Andreas Kaplan. 2019. “A Brief History of Artificial Intelligence: On the Past, Present, and Future of Artificial Intelligence.” California Management Review, vol. 61, no. 4 (July 17, 2019), pp. 5–14. <https://doi.org/10.1177/0008125619864925>, accessed August 29, 2023.
Maslej, Nestor, Loredana Fattorini, Erik Brynjolfsson, John Etchemendy, Katrina Ligett, Terah Lyons, James Manyika, Helen Ngo, Juan Carlos Niebles, Vanessa Parli, Yoav Shoham, Russell Wald, Jack Clark, and Raymond Perrault. 2023. “The AI Index 2023 Annual Report,” AI Index Steering Committee, Institute for Human-Centered AI, Stanford University, Stanford, CA, April 2023. <https://aiindex.stanford.edu/report/>, accessed August 29, 2023.
MEXT (Ministry of Education, Sports, Culture, Science, and Technology, Japan). 2016. Fifth Science and Technology Basic Plan (2016–21). Webpage <https://www.mext.go.jp/en/policy/science_technology/lawandplan/title01/detail01/1375311.htm>, accessed September 14, 2023.
Moor, James. (2006) “The Dartmouth College Artificial Intelligence Conference: The Next Fifty Years.” AI Magazine, Vol. 27 No. 4 (Winter 2006), pp. 87–91.
Richwine, Lisa, and Dawn Chmielewski. May 2, 2023. “Hollywood Writers Strike over Pay in Streaming TV ‘Gig Economy.’” Reuters. <https://www.reuters.com/lifestyle/hollywood-writers-studios-stage-last-minute-talks-strike-deadline-looms-2023-05-01/ >, accessed August 31, 2023.
Schwab. Klaus. 2015. “The Fourth Industrial Revolution: What It Means and How to Respond.” Foreign Affairs, December 12, 2015. <https://www.foreignaffairs.com/world/fourth-industrial-revolution>, accessed August 23, 2023.
Schwab, Klaus. 2016. The Fourth Industrial Revolution. New York: Crown Business, c. 2016. ISBN 1524758876, 9781524758875, 978-1-5247-886-8.
Watanabe, Yasuaki, and Richard Dasher. 2020. “On the Progress of Industrial Revolutions: A Model to Account for the Spread of Artificial Intelligence Innovations across Industry.” Kindai Management Review, vol. 8 (2020), pp. 113–123. The Institute for Creative Management and Innovation, Kindai University (ISSN: 2186-6961).
Zao-Sanders, Marc, and Marc Ramos. 2023. “A Framework for Picking the Right Generative AI Project.” Harvard Business Review Digital, March 29, 2023. Available from HBR.org. Reprint H07J5S.